Beyond Boundaries: Navigating Aerospace
1.
Design and Development of Virtual Sensors for Aero-Engine Test Environments
- A powerful web-based Digital Twin specifically designed to enhance the capabilities of Gas Turbine Research Establishment(GTRE), a subsidiary of DRDO.
- With DataCivet, we’ve harnessed data-driven algorithms to create a versatile solution that addresses sensor failures in aero-engine test environments.
Virtual Sensors, the software representations of physical sensors, offer cost-effective solutions with improved reliability and indirect measurements. DataCivet takes a breakthrough approach to virtual sensor creation that aggregates data from multiple sensors to overcome failures. Our focus is on demonstrating these digital twins’ potential in aerospace testing.
What does it offer?
It can handle diverse parameters like temperature, pressure, vibration, and more across 600 sensors, adapting to variable sampling rates and tough conditions
Strain gauges safeguard fan blades during engine tests. Our virtual sensors mirror physical sensors using real-time and historical data, backed by analytical models even in cases of multiple sensor failure.
It predicts values through imputation, ensuring you’re equipped even when actual data is scarce
What if the physical sensors in an aero-engine test setup stop working? We’ve combined modern deep learning with traditional machine learning to meet this problem. The system is tested with 50 strain sensors running at high speeds (54,000 samples per second) and also with over 1000 sensors of different types, each working at its own speed. This system not only works well in these specific cases but also simplifies the overall sensor setup, needing fewer sensors.
Our tool comes with an easy-to-use interface. You can train and assess the system’s models using this tool. You can also improve the models over time without starting from scratch.
The acquired data can be processed in real-time, removing noise, filtering out unnecessary parts, and reducing the amount of data to make it easier to work with. The system predicts which sensors are failing using a custom-built system that’s trained to understand these situations in real-time.
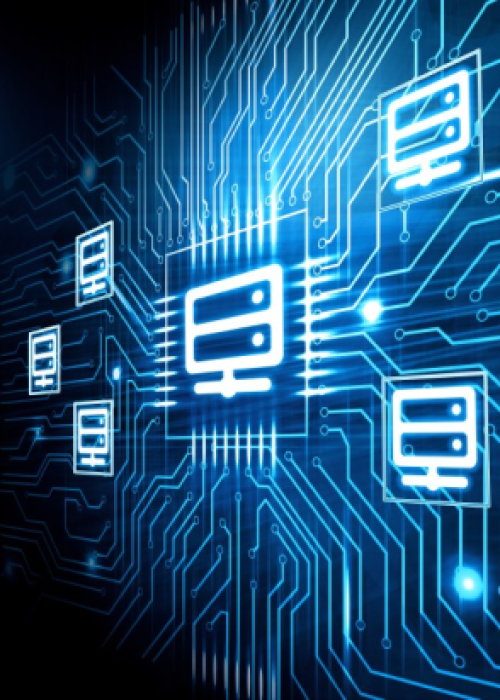
- Data Synchronisation from multiple sources(different acquisition systems)
The synchronisation of data measured at different sampling frequencies (whose RMS trend is generated) is performed by eliminating the initial time delay and using the speed data as a reference.
- Virtual Sensors
Limited sensor placement due to engine complexity, especially in hot zones, can lead to sensor failures in extreme conditions. The data-driven models, called virtual sensors, mitigate sensor failures.
Noise and Outlier Removal
The raw data acquired through multiple data acquisition systems (DAS) from the installed sensors in an aero-engine may contain noise and missing data. The signals can be affected by external factors.
- Automatic Detection of Failed Sensors
The manual inspection of offline sensor data can be avoided by devising an algorithm to detect failed sensors. The incorporation of the sensor failure detection algorithm with the virtual sensor will enable it to be integrated with real-time engine testing.
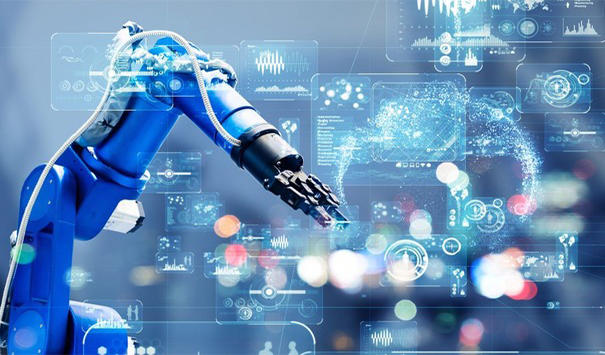
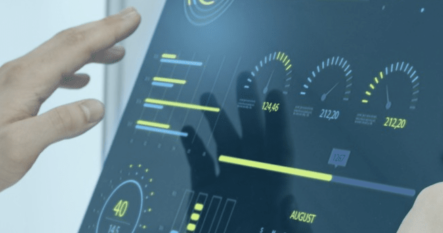
Standardized Report Generation
Generated graphs and plots with observation need to be reported in a standard format. The graphs and correlation maps of strain sensors, among other data representations, need to be automatically generated and captured in a standard reporting format.
Order Tracking
Frequency domain analysis of raw stain signal to detect the frequency components in the signal with the help of order information provided by the subject matter expert. This is required for the scientist to study the signal when resonance occurs in compressor blades.
2.
Digital Twin of Gerotor Pump
Creation of a digital twin of a gerotor pump by using a combination of sensors, software, and analytics.
Real-time monitoring of the pump’s operation, allowing for the identification of potential issues before they become critical.
This synthesis crafts a high-fidelity pump model capable of simulating diverse operational conditions. This innovative process involves gathering real-world data from the physical pump, subjecting it to thorough analysis, and constructing a virtual model that mirrors its behaviour.